- Micro Learning
- Starting with internal AI adoption: the AI Adoption Checklist
Starting with internal AI adoption: the AI Adoption Checklist
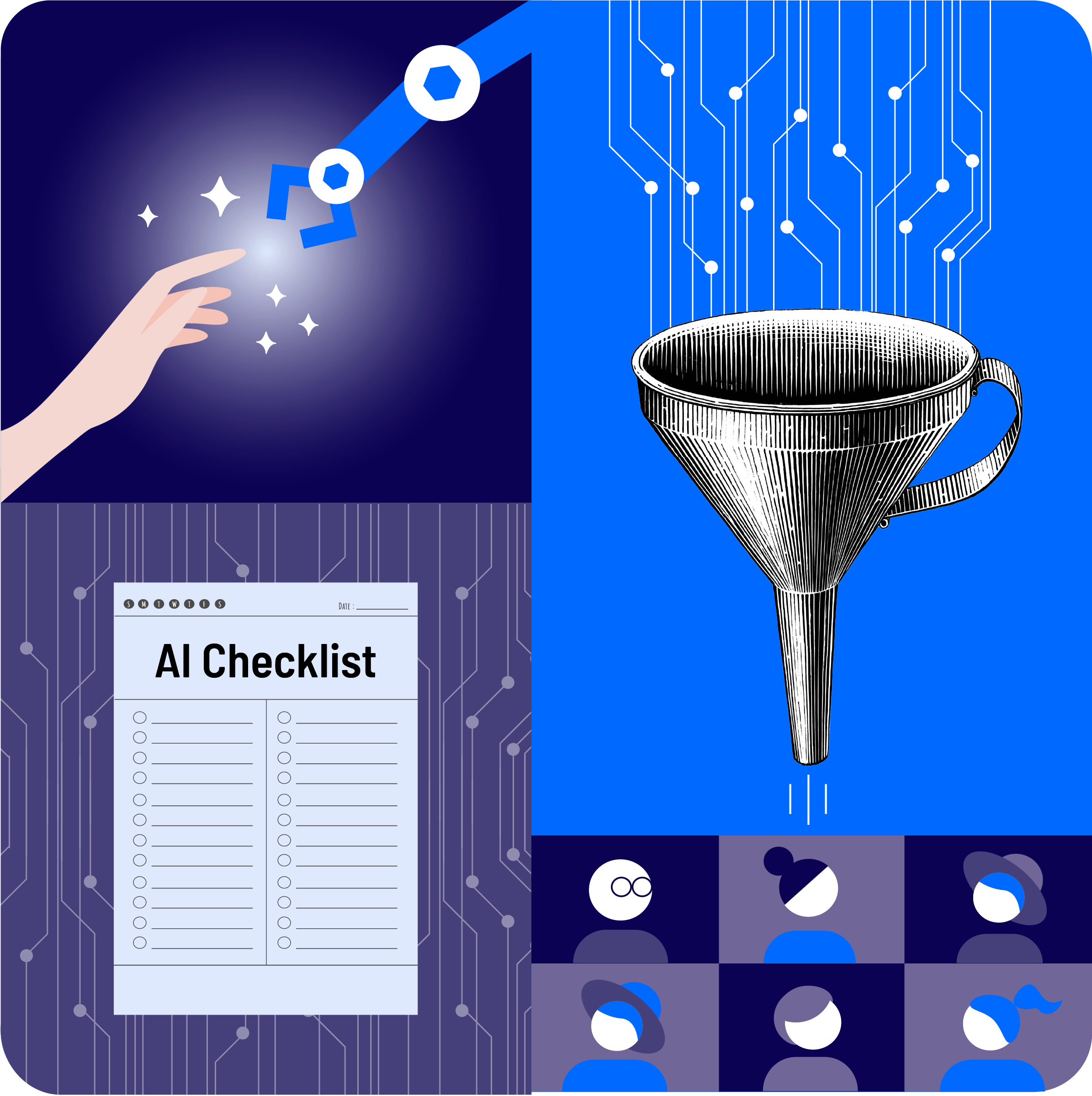
‘Starting your (first) AI project’, could very well be one of your professional New Year’s resolutions (and if it wasn’t yet, it probably is now). But how do you get started? What are the very first steps you need to take to successfully launch an AI pilot project? In this microlearning we will take you by the hand: you will learn to check the very first action points off your list when starting with internal AI adoption. You can find the downloadable AI Checklist at the bottom.
Hi, I’m Thomas. Connect with me on Linkedin to share ideas and keep the conversation going!
This might not come as a surprise, but AI is more popular than ever before. According to the IBM Global AI Adoption Index 2023, companies have been taking serious steps to deploy AI in their business. Approximately 42% of large companies surveyed have actively integrated AI into their operations. Another 40% are in the process of exploring or experimenting with AI, though they haven't yet implemented their models.
|Apart from all the fun we have with AI on the work floor, there are serious drivers behind internal AI adoption (see the image below).
Source: IBM Global AI Adoption Index 2023
At the same time, self-reflection on AI initiatives is important. AI seems at the peak of the hype cycle, and some innovations deserve particular attention.
Source: Gartner Hype Cycle for Artificial Intelligence, 2023
Internal AI adoption doesn’t only come with picking the right type of use case. Being able to remove barriers to adoption is crucial to ensure success. ‘Data Suggests Growth in Enterprise Adoption of AI is Due to Widespread Deployment by Early Adopters, But Barriers Keep 40% in the Exploration and Experimentation Phases’.
One thing is clear: AI is here to stay, and therefore it’s key to learn how AI can be leveraged in a feasible, profitable and ethical way. But with all of these barriers in mind, early adopters might get demotivated quickly.
While starting your first AI project seems tempting, you might want to consider avoiding just launching a project for the sake of joining the hype. Still, you want to start somewhere and, if possible, start an AI project which generates some form of value for your organisation. And yet again, where to start and how to pick your use case?
But don’t worry. By the end of this Microlearning, you have a clear understanding of which actions to take and in which order.
The AI Adoption Checklist
Let’s get it going! In this Microlearning we will study the AI Adoption Checklist. Let’s briefly have a look at the points of the checklist, and further down below, you will learn per action point what you can consider in more detail:
- Assess AI Maturity: assess your team's and organisation's AI experience and readiness.
- Form an AI Pilot Team: Create a small, skilled team considering diverse abilities and mindsets.
- Learn AI Basics: As a team, study fundamental AI and Machine Learning concepts.
- Identify AI-Enhanced Processes: Examine business processes, pinpoint bottlenecks or opportunities, and prioritise them for AI solutions.
- Define Problem Statement: Craft a clear AI use case problem statement, including success criteria, and evaluate AI's suitability versus non-AI options.
- Brainstorm AI Solutions: Generate AI solution ideas and select the most viable one.
- Identify common pitfalls: Investigate common AI implementation pitfalls and strategies to avoid them.
- Implement and Assess: Execute the AI solution experimentally and evaluate success against predefined criteria.
- Prepare for Adoption: After meeting pilot success criteria, plan for broader internal adoption. Also, determine the level of human involvement in the AI process.
- Segment Adoption groups: Identify different adoption groups within your organisation (innovators, early adopters, etc.).
- Tailor Strategies per segment: Develop customised strategies for each adoption segment to facilitate smoother AI integration.
Now, let’s have a look at these action points in more detail:
1. Assess AI Maturity: Assess your team's and organisation's AI experience and readiness.
Evaluate the current understanding and experience your team and organisation have with AI. Consider factors like previous AI projects, familiarity with AI concepts, and technical infrastructure. Rate your AI maturity on the following scale to better align future training and resource allocation.
- AI- Aware: Your team/organisation recognises the importance of AI and conversations are happening.
- AI-Active: Your team is spinning some Proof of Concepts and prototypes, but nothing is in production yet.
- AI-Operational: Some models are in production for important business operations, but you’re facing challenges.
- AI-Transformational: AI is part of your company’s DNA. It drives your strategic decision-making, has a wide reach, and reshapes the way you work.
2. Form an AI Pilot Team: Create a small, skilled team considering diverse abilities and mindsets.
Select a small, dynamic team (2-5 members) for your AI pilot project. Choose members based on a blend of skills – technical expertise in AI, domain knowledge, and project management skills are crucial. Ensure the team members have a growth mindset and are open to learning and adapting.
3. Learn AI Basics: As a team, study fundamental AI and Machine Learning concepts.
This can be through online courses or internal training /knowledge-sharing sessions. Focus on understanding key concepts like:
- Pin down exactly what Machine Learning (ML) and AI are
- Differentiate between types of ML
- Understand potential business applications of ML
- Approach AI projects effectively
Curious to study these concepts?
Have a look at our module AI and Machine Learning Fundamentals.
4. Identify AI-Enhanced Processes: Examine business processes, pinpoint bottlenecks or opportunities, and prioritise them for AI solutions.
First, clearly define what business objectives you aim to achieve with AI. Next, analyse your current business processes in detail. Identify areas where inefficiencies or bottlenecks exist and pinpoint opportunities where AI could bring improvements. Prioritise the top 3 ideas based on impact, feasibility, and alignment with business goals. For example, give a score between 1-5 for every factor and multiply the scores.
- Impact: 5 is high on business impact, 1 is low on business impact
- Feasibility: 5 is easy on feasibility and implementation, 1 is low on feasibility and implementation
- Low dependency: 5 is low dependency on others and other projects, 1 is high dependency on others and other projects
- Alignment with business goals: 5 is high on alignment with business goals, 1 is low on alignment with business goals
(e.g. 4 on impact x 3 on feasibility x 4 on low dependecy x 5 on alignment = 240 points).
Now, you probably know which idea has the highest score and is most suitable to take to the next step. Good to know: these four factors combined will automatically help you to select a so-called “quick win project”.
5. Define Problem Statement: Craft a clear AI use case problem statement, including success criteria, and evaluate AI's suitability versus non-AI options.
Develop a clear and concise problem statement for the idea from step 4, to consider for your AI use case. Include what you want to solve and the success criteria. Assess if AI is the right solution by considering the following questions:
- Is this task recurrent and predictable? Is there a decision that has to be made over and over again, like giving recommendations?
- Is there already an AI solution out there for this problem, or one that could be tweaked to fit it?
- If there’s no AI solution out there, would it be possible to build an in-house solution?
If at least 2 responses are “Yes”, then it looks like AI could be a good solution.
6. Brainstorm AI Solutions: Generate AI solution ideas and select the most viable one.
Think about what AI tools can be considered to solve your problem (and yes, there’s a world beyond ChatGPT, Bard and Bing Chat). For example, go to futuretools.io and find a few tools you can consider.
7. Identify common pitfalls: Investigate common AI implementation pitfalls and strategies to avoid them.
Research and understand common challenges and mistakes in AI projects. Learn from case studies and expert insights to avoid these pitfalls.
- Not asking the right business questions or setting the right goals
- Avoid by: ensure the AI project is realistic and aligned with business goals
- Unrealistic expectations and failure to experiment
- Avoid by: clearly define what the success of the pilot looks like and communicate this with your team.
- Neglecting data quality and ethics (e.g. too much data complexity)
- Avoid by: filling out the AI use case canvas from the module ‘AI Business Strategy’.
- Forgetting to consider the human aspect of change
- Avoid by: Consider fundamental steps in change management from the module ‘Leading Change Management’
- High pricing of AI tools
- Avoid by: first look for free/cheap AI solutions. Only start paying for more expensive tools once the pilot project has proven its impact.
- Limited AI skills and expertise
- Avoid by: setting up documentation and internal learning sessions to transfer knowledge about AI within your organisation
- The project is too difficult to integrate and scale
- Avoid by: picking a project that scores high on feasibility
- Avoid by: picking a project that scores high on feasibility
8. Implement and Assess: Execute the AI solution experimentally and evaluate success against predefined criteria.
Execute your AI project using an experimental approach with your pilot team members. Decide on who does what and for how long you will run the project. Have regular check-in moments and continuously monitor and evaluate the project against the predefined success criteria. Be ready to iterate and make adjustments as needed.
9. Prepare for Adoption: After meeting pilot success criteria, plan for broader internal adoption.
For broader adoption, clearly define the vision for change. List the business goals AI is expected to achieve. Identify the desired behaviours and skills needed within the organisation. Re-evaluate your team’s AI skill level and plan for any necessary training or hiring. Also, decide the level of human involvement in the AI process. Options include human-in-the-loop (active human participation), hybrid intelligence (collaborative human-AI decision-making), human-on-the-loop (human oversight), or fully automated (human out of the loop).
10. Segment Adoption groups: Identify different adoption groups within your organisation.
Develop specific strategies for each adoption segment. Encourage innovators by giving them time to experiment, help early adopters to share their experiences, demonstrate the value to the early majority, provide ample support to the late majority, and develop strategies to engage the laggards.
11. Tailor Strategies per segment: Develop customised strategies for each adoption segment to facilitate smoother AI integration.
Innovators
Innovators are the first group to adopt new technologies, they are risk-takers and are always eager to try new things.
- Adoption tactic: These risk-takers need access to tools and are encouraged by challenges like Hackathons. Help them share their findings.
Early Adopters
Early adopters are the second group to adopt new technologies. They are more cautious than innovators but are still open to new ideas. Here are some tactics to increase AI adoption among early adopters:
- Adoption tactic: Provide comprehensive technical documentation and tutorials, establish user communities, offer personalized training and support, and leverage testimonials and endorsements to enhance trust and credibility.
Early Majority
The early majority is the largest group of adopters. They are more risk-averse than early adopters but are willing to try new technologies if they see a clear benefit. Here are some tactics to increase AI adoption among the early majority:
- Adoption tactic: Focus on demonstrating clear, practical use cases and productivity benefits, provide extensive training and support to ease learning curves, utilize endorsements from successful adopters for social proof, and prioritize user-friendly, intuitive interfaces and features.
Late Majority
The late majority is more sceptical than the early majority and typically adopts new technologies only after they have been widely adopted. Here are some tactics to increase AI adoption among the late majority:
- Adoption tactic: fostering AI adoption involves presenting detailed case studies and testimonials across various industries, offering risk-free trials or pilots for comfortable technology acclimatization, and emphasizing exceptional customer service and support to address concerns and questions.
Laggards
Laggards are the most sceptical and slowest to adopt new technologies. They may require a lot of convincing and hand-holding to get them on board. Here are some tactics to increase AI adoption among laggards:
- Adoption tactic: Provide personalized training and support for effective tool usage, showcase practical value through case studies and success stories, and highlight the consequences of non-adoption to create a sense of urgency and necessity.
Let's practice!
Use the provided AI checklist to prepare for AI adoption.
Open the file, make a copy, read the instructions and provide input on the 3 slides of the checklist.
Are you stuck? Go back to the Microlearning for more context per question.
Wrapping Things Up
Here are the key points we covered:
AI adoption involves assessing organisational readiness, forming skilled teams, and understanding AI basics to leverage its potential ethically and effectively.
Identifying AI-enhanced processes and defining clear problem statements are critical for successful AI implementation and avoiding common pitfalls.
For broad AI adoption, segmenting organisational groups and tailoring strategies to each, coupled with continuous assessment and adaptation, is key to integration success.
I hope you are all as excited as I am for all the incredible things we will be learning in the near future!
Ciao! See you all in the next piece!
Latest articles
Leveraging Competitor Analysis for Business Growth
Imagine you're back in school, ready to ace a test. You peek over...
Balancing Urgency and Consumer Anxiety in E-Commerce
In today's fast-moving market, understanding how to make your...
Data Quality Quick Audits
As organisations strive to become more data-informed, bad data...
GenAI for managers: Marketing and Sales Tools
As sales and marketing managers, leveraging GenAI tools is no...